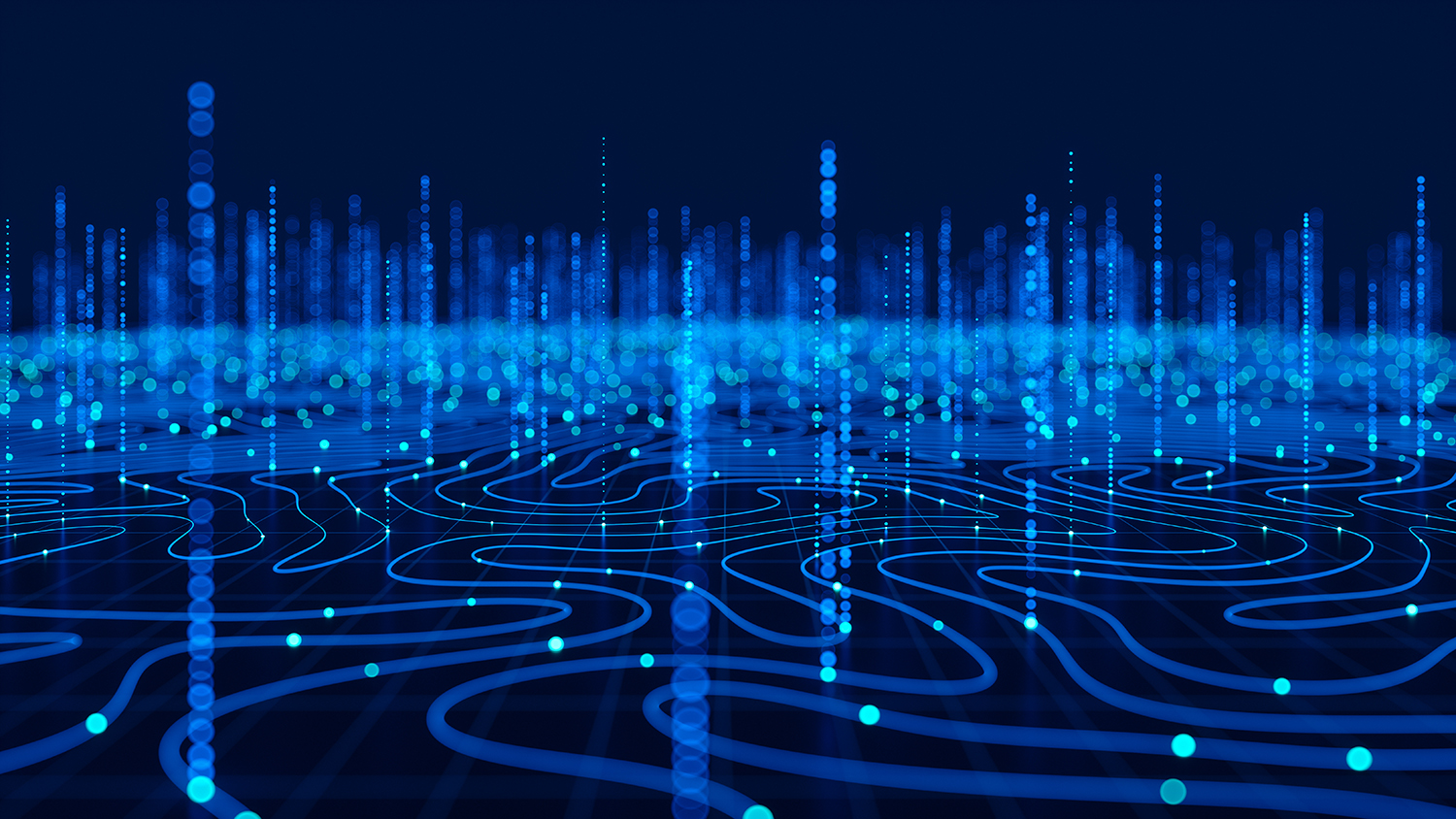
Data is the Lifeblood of AI Models
AI has the potential to improve decision-making, reduce costs, and enhance patient outcomes in the healthcare space. But achieving this potential requires high quality data.
Healthcare data is complex and advances in AI modeling provide more capacity than ever to interpret and predict results from a sea of information. AI models can ingest a variety of data types including:
- Historical and current medical and Rx claims data – diagnosis and prescription fills can be used to predict future risks and healthcare costs.
- Lab data – results may provide leading indicators for new conditions or improvements.
- EHR data – provides emerging information and context for claims which may support early detection of risks.
Data quality matters.
Errors, missing data, and inconsistencies between development and production data sets can lead to low quality predictions. It’s critical to understand new data sources and establish quality monitoring for their use. When assessing and deploying new data sources:
- Assess the density of the data. Is the prevalence of conditions like cancer and heart disease in line with industry averages?
- Assess the distribution of elements like age and gender. Do you see an unusual volume of Medicare aged members when assessing a traditional commercial health plan population?
- Review aggregate results and segments. Do your claim cost amounts make sense for the type of population you’re working with?
- Discuss any critical nuances with the data provider. Are you working with paid claims, allowed claims, billed charges, Medicare allowed amounts, or something else?
- For production deployments, establish protocols on data inputs and predictive outputs to monitor the quality of the data pipeline and any downstream predictions.
Inconsistency in data quality can lead to incorrect health risk predictions ultimately affecting patient outcomes, healthcare costs, and underwriting results.
Just maintaining the data pipeline isn’t enough to deliver quality predictions. Well maintained data pipelines are not immune to industry level events. The organizations responsible for maintaining and managing AI models must be aware of how industry events can influence their data sources and the predictions of their models.
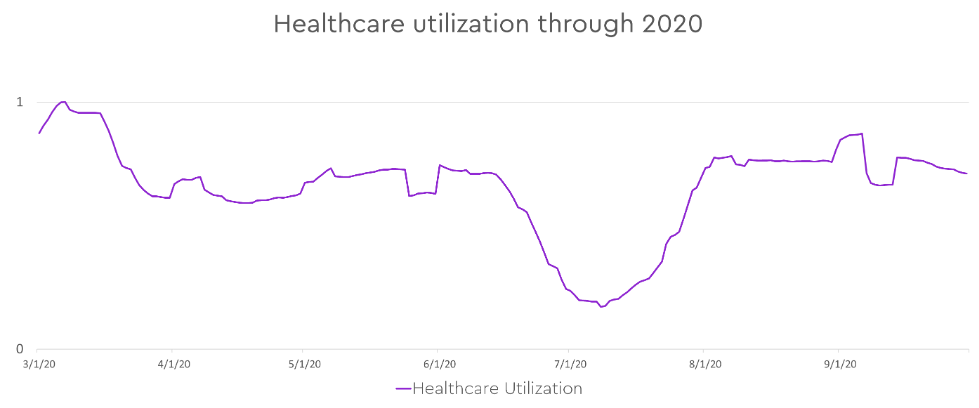
The impact of Covid on healthcare consumption in 2020 is a familiar example where a drop in utilization created challenges for risk takers and models powered by claims data. The health of the population didn’t suddenly improve, and yet consumption of healthcare services temporarily dropped dramatically. Exhibit from a Prealize utilization case study, 2020.
Legacy predictive model architectures may have little capacity to predict from beyond the most recent 12 months of claims data due to the sheer volume of information available in claims. These types of models can be reactive to data gaps like what was seen during Covid and other large industry impacts to data quality. Emerging AI model architectures, like transformer foundation models, can meaningfully use millions of parameters and ingest years of healthcare data overcoming most of the limitations of older model types.
Advances in AI and Predictive modeling technology are enabling rapid improvements across healthcare and risk management. Data is the foundation those improvements are built on.
Choosing the right AI partner for your healthcare financial risk management and care and condition management needs can unlock new opportunities. At Prealize Health we have experts in data engineering, data science, and insurance allowing us to build and maintain high performing models for the past decade. There is no substitute for maintaining quality data pipelines and Prealize Health makes it easy for our clients.
Our team of experts serves organizations looking for the next level of AI powered risk assessment in new business and renewal underwriting. We offer the tools to drive improved financial results, better outcomes in care and condition management, and higher engagement in those programs.
About Us
Founded by two industry thought leaders from Stanford University and through continuous partnership with Stanford, Prealize is a leading provider of AI-driven predictive analytics and insights to health plans, specialty care management companies, healthcare technology companies, employers, and providers across the nation. Committed to transforming healthcare from reactive to proactive, reducing healthcare costs and enabling more people to live healthier lives, Prealize partners positively influence the health trajectory of millions of people.
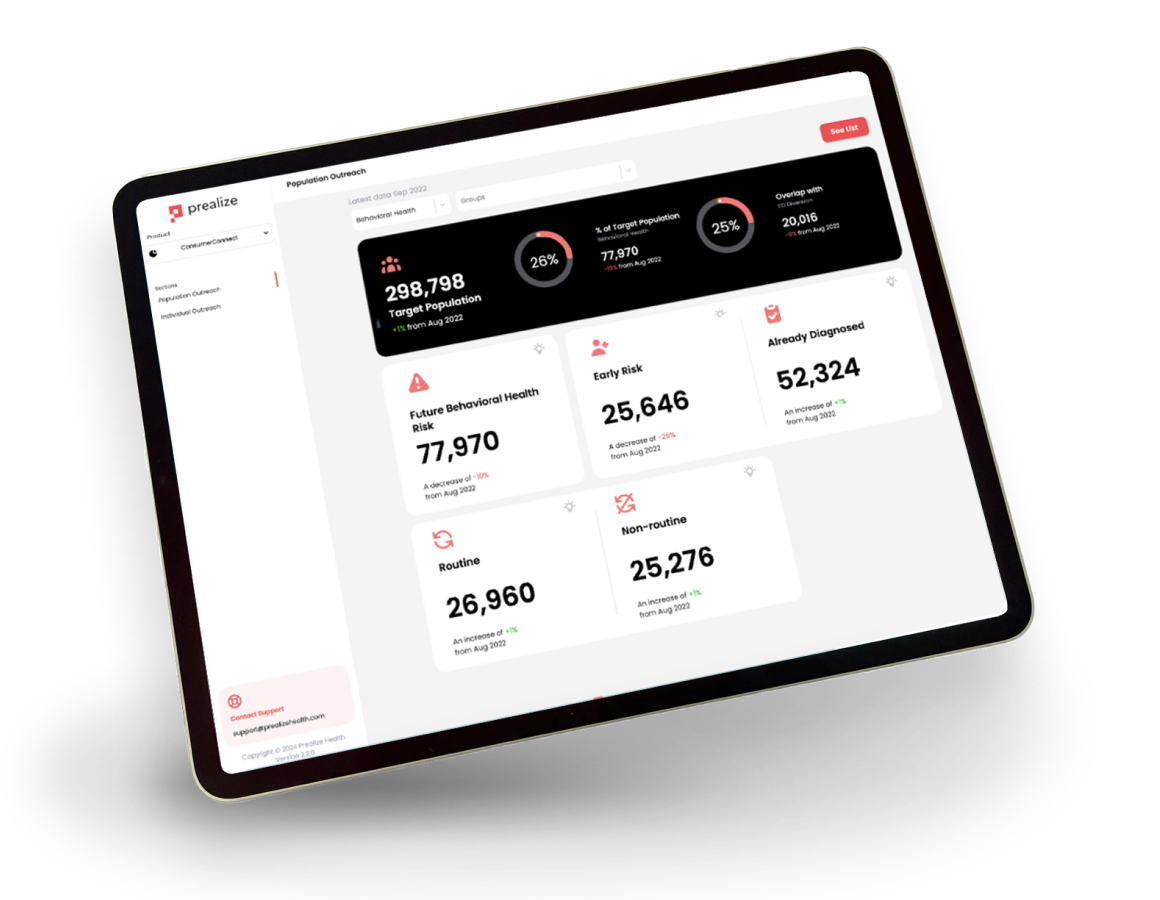
Experience the Prealize Difference
We invite you to experience the transformative power of unparalleled accuracy. Request a demo today and see how Prealize can empower your organization to achieve better health outcomes, reduced costs, and a new era or proactive care.